Project: W20perf - Performance Development of a Medium Speed Diesel Engine
Contact: Senior Research Scientist Raimo Turunen
Email: raimo.turunen@hut.fi
Tel. +358-9-451 3455
The power density and efficiency of diesel engines have been
continuously increasing, while emission reduction efforts are
always targeted on not penalising either of these. These
performance characteristics can nowadays, in principle, be
predicted by modern calculation methods. In this project two
modelling techniques 1) CFD and 2) neural network modelling are
used in analysis and development of the performance
characteristics of a medium speed diesel engine. The example
engine in the project is Wärtsilä 20. CFD (computational fluid
dynamics) is nowadays a popular instrument in examining engine
combustion whereas neural network modelling has proved successful
in analysing systematically large amounts of data in many areas
of science. In this project, the neural network is used to
organize the huge quantity of engine test results relating to
nozzle design, injection advance, combustion chamber shape, etc.
The objective of the project is to serve as a practical test
for these two methods and their application in diesel combustion
analysis. The optimization target was set by specific fuel
consumption at 100%, smoke emission at 10% engine load engine
load and NOx emission according to ISO 8178, E2 cycle.
Results analysis are verified by engine experiments whenever
possible.
The project has got funding granted by TEKES, the Technology
Development Agency of Finland (Engine Technology Programme
ProMOTOR), Wärtsilä Technology and Fortum Oil and Gas. The
project was started in 1998. The analysis tools have been
evaluated; the last verifying engine tests will be done in 2001.
Generally, CFD analysis in the project was succesful although
plenty of engine test data were needed for the tuning of the
model. However, the simulation of internal EGR was found
difficult in connection to the smoke and NOx analysis.
The use of a trained network was very fast. The drawback of
this kind of neural model is that they cannot predict anything
they have not learned. The neural network analysis showed that
there are no better alternatives than those which had already
been found in the original tests by the engine manufacturer,
although it might have been possible to produce similar results
with different configurations.
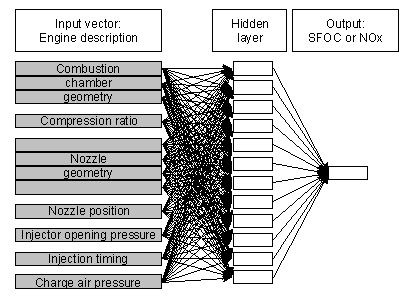
Fully connected feed-forward net of multi-layer perceptron (MLP)
type of a diesel engine.
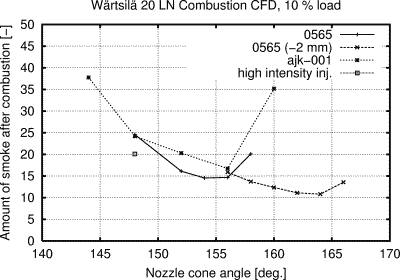
CFD forecasts of smoke characteristics with different
injection and combustion chamber configurations
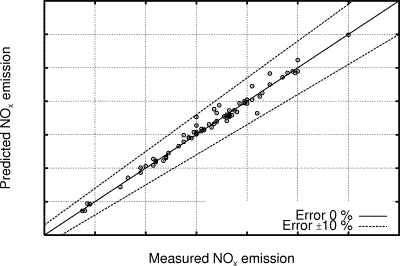
NOx emission response of the neural model compared to engine
test results
|